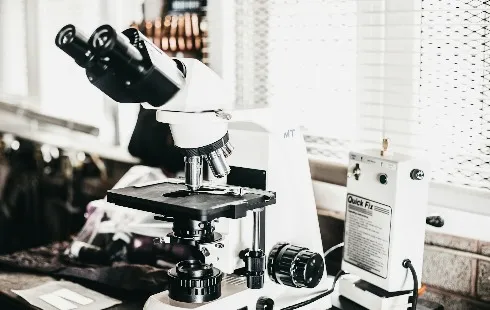
Gonadorelin Peptide: A Gateway to Understanding Endocrine Dynamics
Section: Science
Identifying whether thyroid nodules are benign or malignant prior to surgical intervention has long posed a challenge in the medical field. However, recent research indicates that an innovative combination of artificial intelligence (AI) and advanced data analysis techniques may significantly enhance the accuracy of cancer predictions.
This groundbreaking study was conducted by a team from Cornell University's Ann S. Bowers College of Computing and Information Science and the Icahn School of Medicine at Mount Sinai. The researchers developed machine learning models designed to detect thyroid cancer through the analysis of ultrasound images, employing a method known as topological data analysis (TDA). This approach captures intricate shape and pattern details from ultrasounds, demonstrating a marked improvement over traditional methods that rely solely on basic ultrasound features for cancer prediction.
Should these findings be validated through larger-scale studies, the integration of this method with conventional risk assessment techniques could provide enhanced guidance for patients and potentially reduce the number of unnecessary surgeries performed.
Denise Lee, a senior author of the study, emphasized the importance of this research in refining preoperative counseling for patients and assessing cancer recurrence risks. The study, titled 'A Proof-of-Concept Investigation into Predicting Follicular Carcinoma on Ultrasound Using Topological Data Analysis and Radiomics,' was published in the journal Imaging.
As the incidence of incidentally discovered thyroid nodules rises due to increased routine imaging, the traditional risk categorization process often involves ultrasound examinations, fine needle aspiration biopsies, and molecular testing. Unfortunately, many cases yield ambiguous results, resulting in up to 25% of patients undergoing surgery for benign conditions.
Lee noted the subjective nature of ultrasound interpretation, which complicates diagnosis. The objective of this research was to determine if AI and TDA could standardize ultrasound features to yield more reliable predictions.
Previous attempts to predict cancer using ultrasound images have often relied on deep learning models that function as 'black boxes,' producing predictions based on unknown ultrasound features without clear explanations. In contrast, TDA allows researchers to trace identified features back to the ultrasound images themselves.
Andrew Thomas, the first author of the study, pointed out that the features utilized in this research are relatively interpretable. They can be assessed similarly to other measurable characteristics, such as the quantity of calcifications or the irregularity of the thyroid's borders observed in ultrasound images, all of which are captured through TDA.
TDA operates by converting grayscale ultrasound images into a series of binary black and white images. Starting with the darkest pixel, the method progressively raises a threshold, causing lighter pixels to change to black until dark masses merge, while lighter areas gradually diminish. This sequence provides valuable information about the connectivity of various thyroid regions, which can be indicative of an individual's risk of cancer.
The results of the study showed a 91% sensitivity in detecting cancer when present and a 71% specificity in accurately identifying benign nodules. In comparison, models that did not utilize TDA achieved considerably lower accuracy, with only 43% success in correctly identifying true benign cases. Furthermore, TDA models outperformed several previous attempts that relied on deep learning.
Currently, the research team is expanding this methodology to a larger dataset of thyroid ultrasounds to further investigate its application in cancer risk predictions. They anticipate that this approach could eventually be adapted for use in other medical imaging contexts and various cancer types.
In a related effort, Thomas and Matteson have successfully employed TDA to assess dynamics in microscopic images of metal nanoparticles enhanced by deep learning.
Overall, the findings underscore the potential for statistical methods to reveal consistent shape-related features that may vary across different patients, enhancing the understanding of cancer risks.
Section: Science
Section: Health
Section: Arts
Section: Health
Section: Science
Section: News
Section: News
Section: Health Insurance
Section: Health
Section: News
Health Insurance in Germany is compulsory and sometimes complicated, not to mention expensive. As an expat, you are required to navigate this landscape within weeks of arriving, so check our FAQ on PKV. For our guide on resources and access to agents who can give you a competitive quote, try our PKV Cost comparison tool.
Germany is famous for its medical expertise and extensive number of hospitals and clinics. See this comprehensive directory of hospitals and clinics across the country, complete with links to their websites, addresses, contact info, and specializations/services.
The granddaughter of Claire Zachanassian makes a return to Güllen, the impoverished hometown of her late grandmother, for a performance. Having never fully engaged with her grandmother's past, she is eager to finally discover Güllen. The sound of her last name stirs the entire town into action.
No comments yet. Be the first to comment!