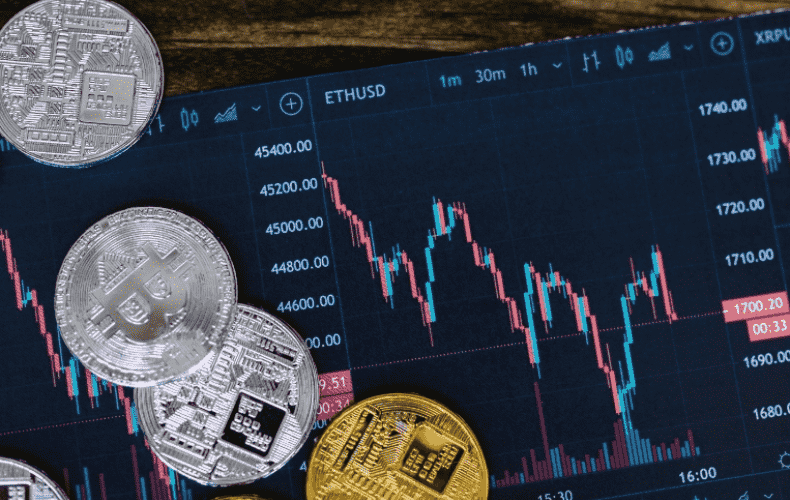
Crypto Investment Entry and Exit Strategies of Institutional Investors
Section: Business
Magnetic Resonance Imaging (MRI) is a vital non-invasive imaging technology used to visualize intricate structures within the human brain. Utilizing a combination of magnetic fields and radio waves, MRI produces detailed images of soft tissues without exposing patients to harmful radiation. However, traditional MRI techniques are not without challenges.
One of the main issues affecting image quality is participant movement during scans. Actions such as blinking, breathing, and other involuntary movements can lead to blurred images or the creation of ghost artifacts, complicating the diagnosis and research of neurological conditions. This is particularly problematic in pediatric patients who may find it challenging to remain still throughout the procedure. Given the significance of MRI in clinical evaluations, maintaining high image quality is essential.
In response to these challenges, a team of researchers led by an associate professor at the University of North Carolina at Chapel Hill School of Medicine has developed a novel model aimed at enhancing the quality of brain MRI scans. This foundation model, referred to as the Brain MRI Enhancement foundation (BME-X), is capable of executing various functions, including motion correction, super-resolution, noise reduction, harmonization, and improving imaging contrast. The findings of this research have been published in a reputable scientific journal.
According to the lead researcher, the quality of imaging plays a critical role in accurately visualizing brain anatomy and pathology, which in turn informs clinical decision-making. The BME-X model allows for more precise and dependable analyses of brain structures, which is crucial for the early detection, diagnosis, and monitoring of neurological disorders.
The BME-X model underwent extensive testing, analyzing over 13,000 images from a variety of patient demographics and MRI scanner types. The results demonstrated that it surpassed existing advanced methods in correcting for body motion, reconstructing high-resolution images from their lower-resolution counterparts, reducing noise, and managing pathological MRI images effectively.
A particularly noteworthy feature of this model is its ability to harmonize images obtained from different MRI machines. With numerous MRI scanners in use across healthcare facilities, each employing distinct models and imaging parameters, variability in the results can pose significant challenges for clinicians and researchers. The BME-X model can standardize data from various sources, yielding harmonized results that facilitate both clinical applications and research endeavors.
This capability to harmonize imaging data holds potential benefits for clinical trials and studies that encompass multiple research institutions. By aiding the establishment of standardized imaging protocols and practices in neuroimaging, the BME-X model could streamline research processes and enhance the reliability of results across diverse settings.
In conclusion, the introduction of the BME-X model represents a significant advancement in the field of MRI technology. Its ability to improve image quality through motion correction, noise reduction, and data harmonization positions it as a valuable tool for both clinical and research applications in neurology. As the medical field continues to evolve, innovations like BME-X will play a crucial role in enhancing diagnostic accuracy and improving patient outcomes.
Section: Business
Section: Arts
Section: Arts
Section: Business
Section: Business
Section: Arts
Section: Health
Section: Arts
Section: News
Section: News
Health Insurance in Germany is compulsory and sometimes complicated, not to mention expensive. As an expat, you are required to navigate this landscape within weeks of arriving, so check our FAQ on PKV. For our guide on resources and access to agents who can give you a competitive quote, try our PKV Cost comparison tool.
Germany is famous for its medical expertise and extensive number of hospitals and clinics. See this comprehensive directory of hospitals and clinics across the country, complete with links to their websites, addresses, contact info, and specializations/services.
One of the most beautiful squares transforms into a summer stage every year for two days. The Gärtnerplatz Open-Air features a free music and cultural program across three stages, as well as street food from local vendors. On Saturday, the main stage at Gärtnerplatz offers something for everyone,...
No comments yet. Be the first to comment!