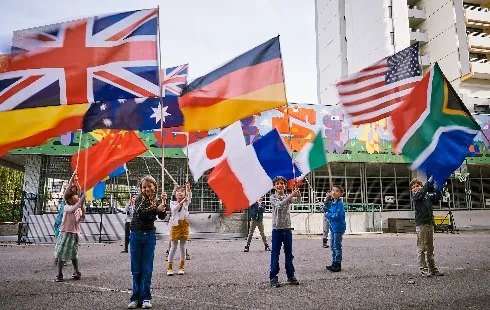
Discover the Future of Learning at Bavarian International School's City Campus Open Day
Section: News
Researchers at the University of Chicago have developed an innovative brain network model capable of predicting when individuals will experience surprise. This groundbreaking study, published in Nature Human Behaviour, demonstrates that the model can accurately gauge surprise across various contexts, including task performance and video viewing.
Surprise is a fundamental human emotion triggered when actual experiences deviate from expectations. Previous psychological research has explored the underlying neural mechanisms of this response. The current study builds on earlier findings that indicate common brain activity patterns associated with the experience of surprise.
The primary aim of the research was to investigate whether brain activity could serve as a predictive tool for surprise across different scenarios. By analyzing brain activity patterns, the researchers sought to determine if they could accurately anticipate moments of surprise in individuals engaged in diverse activities.
Utilizing functional magnetic resonance imaging (fMRI), the researchers examined several datasets previously collected by other research groups. One dataset involved participants learning to predict the location of a cartoon bag containing coins on a computer screen. When the bag appeared unexpectedly, it was assumed that the participants experienced surprise.
In another dataset, volunteers watched videos of basketball games. The level of surprise was measured based on unexpected changes in their expectations of the game's outcome. The final dataset featured fMRI data from participants watching animated cartoons with varying levels of surprising character actions.
To predict surprise across these distinct scenarios, the researchers created a brain network model known as the surprise edge-fluctuation-based predictive model (EFPM). This model tracks the dynamic interactions between different brain regions to forecast when individuals are likely to experience surprise.
The researchers first identified brain interactions that indicated surprise during the learning task. They then sought to determine if these interactions could also predict surprise in participants watching basketball games and cartoons.
The findings revealed that the model effectively predicted surprise moments for individuals in various contexts, suggesting that certain brain regions respond similarly to expectation violations, regardless of the specific task or situation.
Although the predictions were not flawless, they were significantly more accurate than random chance. This research highlights the potential for the model to extend beyond predicting surprise, suggesting that it may also be applicable in forecasting other emotional responses, such as attention levels or happiness.
The implications of this study may pave the way for future research aimed at predicting human emotions based on recorded brain activity. By utilizing datasets from other researchers, the study underscores the importance of open science and data sharing within the scientific community.
Looking ahead, the researchers plan to explore the model's predictive capabilities in an even wider array of contexts, including storytelling, music appreciation, and social interactions. They are also interested in examining how individual differences in surprise relate to memory and learning outcomes.
This research not only advances our understanding of the neural basis of surprise but also emphasizes the shared neural signatures of emotional responses across different people and situations.
Section: News
Section: Health
Section: News
Section: News
Section: Travel
Section: News
Section: News
Section: Politics
Section: Arts
Section: News
Health Insurance in Germany is compulsory and sometimes complicated, not to mention expensive. As an expat, you are required to navigate this landscape within weeks of arriving, so check our FAQ on PKV. For our guide on resources and access to agents who can give you a competitive quote, try our PKV Cost comparison tool.
Germany is famous for its medical expertise and extensive number of hospitals and clinics. See this comprehensive directory of hospitals and clinics across the country, complete with links to their websites, addresses, contact info, and specializations/services.
Experience the extraordinary talent of Evgeny Kissin, a pianist who seamlessly merges with the music, producing a sound that resonates with raw power. Renowned as a genius pianist and a virtuosic musician, Kissin embodies the captivating union of the artist with his instrument, showcasing the...
No comments yet. Be the first to comment!