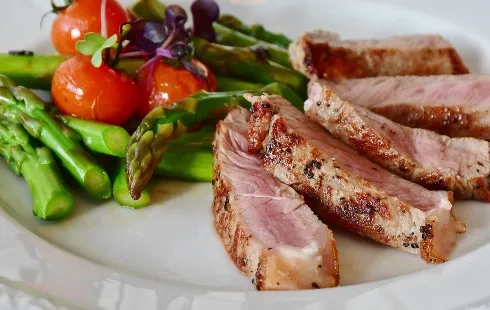
Prioritizing Food Quality Over Carb or Fat Restrictions for Heart Health
Section: Health
The increasing complexity of medical imaging poses significant challenges for healthcare professionals tasked with diagnosing diseases. For example, conditions like pleural effusion, characterized by fluid accumulation in the lungs, can easily be mistaken for pulmonary infiltrates, which involve pus or blood accumulation. To aid in the diagnostic process, artificial intelligence (AI) models can assist clinicians by identifying subtle patterns and improving diagnostic efficiency.
However, traditional AI models often yield a single prediction, which may not encompass the range of possibilities that a clinician needs to consider. A method known as conformal classification allows for the generation of a set of potential diagnoses, enhancing the decision-making process. Yet, one of the drawbacks of this approach is that it can produce overly large sets of predictions, making it cumbersome for clinicians.
Researchers at the Massachusetts Institute of Technology (MIT) have introduced a novel enhancement to conformal classification, aiming to reduce the size of these prediction sets by up to 30% while simultaneously improving reliability. A more compact set of predictions can help clinicians focus on the most relevant diagnoses, potentially expediting patient treatment.
Divya Shanmugam, a researcher involved in this study, emphasizes that a smaller prediction set improves informativeness without sacrificing accuracy. This advancement could be applicable in various classification scenarios, such as wildlife identification, where the goal is to narrow down options efficiently.
In high-stakes environments like medical diagnostics, AI systems typically provide a probability score along with each prediction, indicating the model's confidence level. For instance, a model might suggest a 20% probability that an image corresponds to a specific diagnosis. However, trust in these confidence scores is often low due to prior research showing their inaccuracy. By utilizing conformal classification, the model replaces a single prediction with a set of the most likely diagnoses, guaranteeing that the correct diagnosis is included within that set.
Despite its advantages, conformal classification can lead to impractically large prediction sets, particularly when dealing with extensive classification tasks, such as identifying numerous animal species from images. The researchers found that the inherent variability in AI predictions can cause the model to generate disparate prediction sets based on minor alterations to the input data, such as image rotation.
To enhance the utility of conformal classification, the research team incorporated a technique known as test-time augmentation (TTA). This method involves creating multiple variations of a single image, including adjustments like cropping and flipping, and then aggregating the predictions from each version. By employing TTA, the researchers improve both the accuracy and robustness of the predictions.
To implement TTA effectively, the researchers set aside a portion of labeled image data for the conformal classification process. They developed an approach to aggregate the augmented data that maximizes the underlying model's predictive accuracy. Subsequently, they applied conformal classification to the newly transformed TTA predictions, resulting in a more concise set of likely diagnoses while maintaining confidence guarantees.
The study found that the combination of TTA and conformal prediction significantly reduced the size of prediction sets, with reductions ranging from 10% to 30% across various standard image classification benchmarks. Notably, this reduction in set size was achieved without compromising the probability guarantees associated with the predictions.
Future research will focus on validating the effectiveness of this methodology in text classification models, while also exploring ways to minimize the computational resources required for TTA.
Section: Health
Section: Arts
Section: Health
Section: Science
Section: News
Section: News
Section: Health Insurance
Section: Health
Section: News
Section: Arts
Health Insurance in Germany is compulsory and sometimes complicated, not to mention expensive. As an expat, you are required to navigate this landscape within weeks of arriving, so check our FAQ on PKV. For our guide on resources and access to agents who can give you a competitive quote, try our PKV Cost comparison tool.
Germany is famous for its medical expertise and extensive number of hospitals and clinics. See this comprehensive directory of hospitals and clinics across the country, complete with links to their websites, addresses, contact info, and specializations/services.
The granddaughter of Claire Zachanassian makes a return to Güllen, the impoverished hometown of her late grandmother, for a performance. Having never fully engaged with her grandmother's past, she is eager to finally discover Güllen. The sound of her last name stirs the entire town into action.
No comments yet. Be the first to comment!