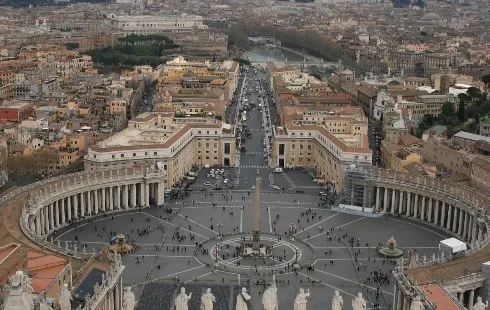
Inside the Conclave: 10 Fascinating Facts About the Secretive Process to Elect a New Pope
Section: Politics
In 2025, significant strides in artificial intelligence (AI) and machine learning are poised to enhance our comprehension of animal communication, addressing a long-standing enigma: the meanings behind animal vocalizations. The recent Coller-Dolittle Prize, which offers substantial financial awards for breakthroughs in decoding animal sounds, reflects a growing optimism within the scientific community that advancements in technology are bringing this ambitious goal closer to reality.
Various research initiatives have been dedicated to developing algorithms capable of interpreting animal sounds. Notably, Project Ceti has been focusing on deciphering the intricate click patterns of sperm whales and the melodic songs of humpback whales. These contemporary machine learning techniques necessitate vast datasets, which have historically been difficult to acquire due to the scarcity of high-quality annotated animal sound data.
For instance, large language models (LLMs), such as ChatGPT, are trained on extensive text datasets from the internet, providing a stark contrast to the limited datasets available for studying animal communication. While LLMs are built on over 500 GB of textual information, Project Ceti's research on sperm whale communication has had access to merely over 8,000 vocalizations. This disparity highlights the challenges researchers face in establishing a comprehensive understanding of animal communication.
Furthermore, the interpretation of human language is grounded in a shared understanding of semantics and syntax. In contrast, scientists often grapple with the ambiguity in animal vocalizations, such as distinguishing the meanings behind various wolf howls. Such complexities complicate the task of determining whether these sounds can be considered analogous to human words.
Nevertheless, 2025 is expected to usher in new developments in both the availability of animal communication data and the sophistication of AI algorithms that will be applied to analyze this data. The rise of affordable recording technologies, such as AudioMoth, has democratized access to high-quality sound capture, enabling research teams to collect extensive datasets by recording animal sounds in their natural habitats around the clock.
As a result, massive datasets are becoming accessible, allowing researchers to analyze the vocalizations of various species, from gibbons in tropical jungles to birds in expansive forests. Automated detection algorithms powered by convolutional neural networks are now capable of processing vast amounts of audio, efficiently identifying and categorizing animal sounds based on their unique acoustic properties.
Once these large datasets are compiled, advanced analytical techniques, including deep neural networks, can be employed to uncover patterns and structures within sequences of animal vocalizations. Such analyses may reveal underlying structures that bear resemblance to human language.
Despite these advancements, a fundamental question persists: what are the ultimate objectives of decoding animal sounds? Some organizations, like Interspecies.io, explicitly aim to translate interspecies communication into comprehensible signals for humans, suggesting an ambitious goal of converting animal sounds into human language. However, there is a consensus among scientists that non-human animals may not possess a structured language akin to that of humans.
The Coller-Dolittle Prize adopts a more nuanced approach, seeking methods to interpret or understand the communicative signals of various organisms. This goal acknowledges the possibility that animal communication may not follow a structured language model, prompting a more exploratory investigation into the nuances of animal interactions.
As humanity progresses towards enhancing our understanding of animal communication, 2025 stands to be a pivotal year. The potential to deepen our insights into the ways animals convey information to one another will undoubtedly reshape the field of animal behavior research and our relationship with the natural world.
Section: Politics
Section: Health Insurance
Section: Health
Section: Health
Section: Politics
Section: News
Section: Business
Section: Science
Section: Politics
Section: News
Health Insurance in Germany is compulsory and sometimes complicated, not to mention expensive. As an expat, you are required to navigate this landscape within weeks of arriving, so check our FAQ on PKV. For our guide on resources and access to agents who can give you a competitive quote, try our PKV Cost comparison tool.
Germany is famous for its medical expertise and extensive number of hospitals and clinics. See this comprehensive directory of hospitals and clinics across the country, complete with links to their websites, addresses, contact info, and specializations/services.
Join us for an exciting theatrical experience on Saturday, May 10, 2025, from 19:30 to 22:00 at the Münchner Kammerspiele - Werkraum. This performance, directed by Melina Dressler, is a directorial exercise inspired by Heiner Müller's 'Quartett' and incorporates texts by Michel Foucault. The...
No comments yet. Be the first to comment!