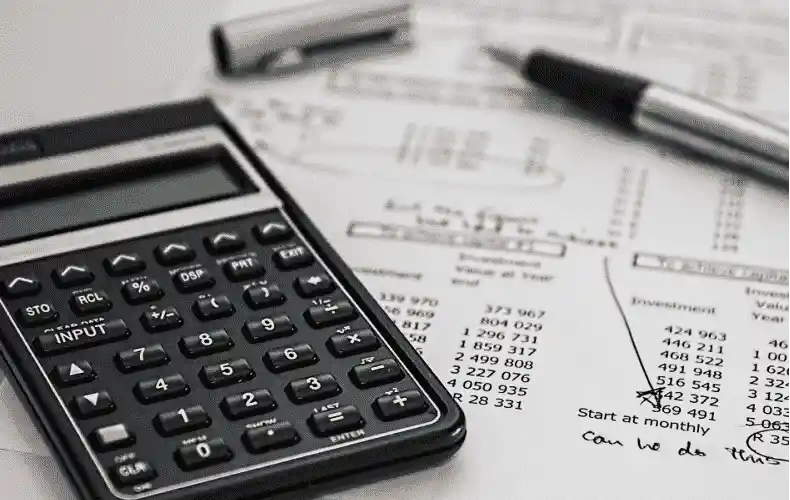
New to Germany? Avoid These Common Health Insurance Mistakes
Section: Health Insurance
Research from York University indicates that the way individuals grasp objects might provide a more straightforward method for diagnosing autism spectrum disorder (ASD). This study, published in the journal Autism Research, highlights the potential of motor patterns to serve as diagnostic indicators for this neurodevelopmental condition.
The study employed machine learning techniques to analyze the hand movements of both autistic and non-autistic individuals during grasping tasks. According to the researchers, their models achieved an impressive classification accuracy of approximately 85% when identifying autism through these motor patterns.
Currently, autism affects around one in every 50 children in Canada, and obtaining a timely diagnosis remains a significant hurdle. The findings from this research contribute to the expanding evidence that subtle motor behaviors may provide critical diagnostic cues, a concept that has not yet been widely utilized in clinical settings.
ASD is characterized not only by social and communication difficulties but also by motor irregularities that often manifest in early childhood. Early detection of these motor patterns could facilitate quicker diagnoses and timely interventions, ultimately improving outcomes for those on the autism spectrum.
Expert collaborators in the study pointed out that while current diagnostic markers mainly focus on behaviors that appear later in development, identifying early motor indicators could significantly reduce the age at which autism is diagnosed.
Young adult participants, both autistic and non-autistic, were involved in the study, employing their thumbs and index fingers--equipped with tracking markers--to grasp and manipulate blocks of different sizes. This approach aimed to eliminate developmental delays as a confounding factor, allowing for clearer comparisons between the two groups.
Results indicated that the research could capture nuanced differences in motor control with over 84% accuracy, revealing distinct kinematic features in the grasping movements of the two groups. This novel analysis of precision-grasping tasks has not typically been part of prior autism research.
The application of machine learning in this context offers researchers new avenues to explore motor patterns as part of autism assessments. The implications of this research could lead to the creation of more accessible diagnostic tools and timely support mechanisms for individuals with autism.
This innovative approach promises to transform how autism is diagnosed, potentially leading to earlier interventions that could significantly enhance the quality of life for those affected by the disorder.
Section: Health Insurance
Section: Health
Section: News
Section: Arts
Section: Arts
Section: Politics
Section: Politics
Section: News
Section: Politics
Section: Health Insurance
Health Insurance in Germany is compulsory and sometimes complicated, not to mention expensive. As an expat, you are required to navigate this landscape within weeks of arriving, so check our FAQ on PKV. For our guide on resources and access to agents who can give you a competitive quote, try our PKV Cost comparison tool.
Germany is famous for its medical expertise and extensive number of hospitals and clinics. See this comprehensive directory of hospitals and clinics across the country, complete with links to their websites, addresses, contact info, and specializations/services.
Offene Wunde is a documentary theater piece about the attack at the Olympia Einkaufszentrum (OEZ) by Tunay Önder and Christine Umpfenbach. On July 22, 2016, a perpetrator motivated by racism killed nine young people from Munich: Armela, Can, Dijamant, Guiliano, Hüseyin, Roberto, Sabine, Selçuk,...
No comments yet. Be the first to comment!