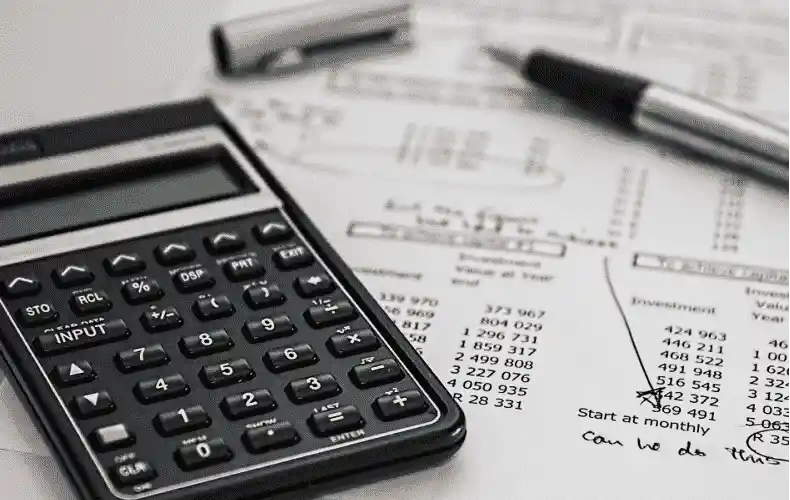
New to Germany? Avoid These Common Health Insurance Mistakes
Section: Health Insurance
Recent advancements in machine learning are set to revolutionize the field of newborn genetic screening. A study from researchers at Mass General Brigham has highlighted notable inconsistencies in gene selection among various genomic sequencing programs for newborns, underscoring the need for a more standardized approach.
The BabySeq Project, initiated over a decade ago, aimed to return genomic sequencing results to parents and evaluate its implications for infant care. Currently, more than 30 international initiatives are assessing the potential of genomic sequencing in newborn screening. However, the study reveals significant variability in the genes selected by these programs.
Published in the journal Genetics in Medicine, the research advocates for a structured, data-driven methodology to prioritize genes for public health initiatives. According to a co-senior author, the thoughtful selection of genes and conditions is crucial for optimizing the benefits of genomic screening.
The researchers introduced a machine learning model designed to enhance the consistency of gene selection in newborn genomic sequencing programs. This model is the first output from the International Consortium of Newborn Sequencing (ICoNS), which was established in 2021. The consortium aims to harmonize efforts in genomic screening across different regions.
In their analysis, the researchers examined 4,390 genes across 27 different newborn sequencing programs. They found that the number of genes included in these programs varied significantly, ranging from 134 to 4,299. Alarmingly, only 74 genes, representing just 1.7% of those analyzed, were included in over 80% of the programs surveyed. The factors most strongly influencing gene selection included whether the condition appears on the U.S. Recommended Uniform Screening Panel, the availability of comprehensive natural history data, and evidence supporting the efficacy of treatment options.
Leveraging these insights, the research team developed a machine learning model incorporating 13 predictive factors, achieving a high degree of accuracy in forecasting gene inclusion across diverse programs. This innovative model produces a ranked list of genes that can adapt to emerging evidence and regional health needs, facilitating more informed decision-making in newborn genomic screening initiatives globally.
This research marks a pivotal advancement in the quest for consistency in newborn genomic screening, aiming to ensure that gene selection aligns with the latest scientific knowledge and public health objectives.
Section: Health Insurance
Section: Health
Section: News
Section: Arts
Section: Arts
Section: Politics
Section: Politics
Section: News
Section: Politics
Section: Health Insurance
Health Insurance in Germany is compulsory and sometimes complicated, not to mention expensive. As an expat, you are required to navigate this landscape within weeks of arriving, so check our FAQ on PKV. For our guide on resources and access to agents who can give you a competitive quote, try our PKV Cost comparison tool.
Germany is famous for its medical expertise and extensive number of hospitals and clinics. See this comprehensive directory of hospitals and clinics across the country, complete with links to their websites, addresses, contact info, and specializations/services.
Davi Pontes and Wallace Ferreira present Repertório N.2 and Repertório N.3. Two young dancers stand completely naked on stage, rhythmically stamping their feet in energetic patterns that continuously evolve. The physical intensity reverberates through the space, with sweat flying and heavy...
No comments yet. Be the first to comment!