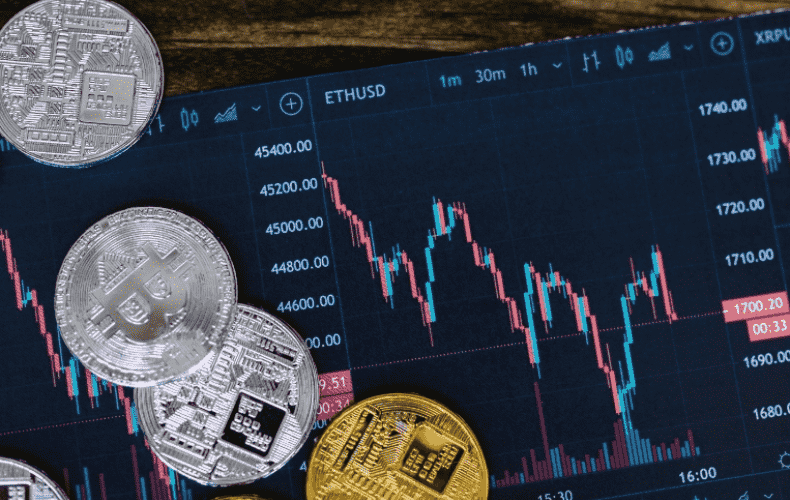
Crypto Investment Entry and Exit Strategies of Institutional Investors
Section: Business
Recent advancements in survival analysis techniques have significantly enhanced the ability to interpret clinical and epidemiological data. The restricted mean survival time (RMST) analysis has gained traction across various fields, including healthcare, over the past 25 years. This method simplifies the understanding of average survival times among patients following diagnosis or treatment, as well as the influencing factors within a defined period.
Challenges with Traditional MethodsUnlike conventional Cox regression models, which operate under the assumption of constant hazard over time, RMST does not rely on this proportional hazard assumption. However, a major limitation of RMST is the difficulty in identifying the optimal threshold time for comparisons between treatment groups. This shortcoming can lead to less statistically powerful outcomes.
New Methodology DevelopedTo overcome this challenge, researchers have introduced a novel methodology utilizing the reduced piecewise exponential model to establish the optimal threshold time for RMST analysis in studies involving two groups. This innovation is particularly crucial in medical research, where the probability of a specific event can vary significantly during different treatment phases.
Research FindingsThe research team calculated the ideal threshold from significant changepoints in hazard rates, comparing their findings with the maximum possible threshold time. The findings, published in the American Journal of Epidemiology, demonstrated the advantages of the new method through multiple simulation studies and real-world applications, including a clinical trial and an epidemiological study.
In their simulations, the new model was used to evaluate Type 1 error rates and statistical power under varying hazard rates for different groups. When compared to the standard logrank test, the new approach yielded superior results.
Real-World ApplicationsIn practical applications, traditional statistical methods failed to reveal significant differences between treatments in both scenarios evaluated. However, the innovative model indicated that one treatment was markedly more effective in each case. The first scenario involved comparing two treatments for patients with non-small-cell lung cancer who exhibited lower levels of a specific biomarker, while the second assessed the time to decline in individuals with mild dementia living with caregivers versus those without.
Future Research DirectionsThese promising results indicate the potential of this new methodology to provide more robust comparisons than existing techniques for analyzing time-to-event outcomes. Further research is necessary to evaluate its effectiveness in studies involving multiple groups and various covariates, such as age, ethnicity, and socioeconomic status.
Collaborative EffortsContributors to this study include doctoral students, distinguished professors, and external collaborators from leading organizations, emphasizing the collaborative nature of this research endeavor.
Section: Business
Section: Arts
Section: Arts
Section: Business
Section: Business
Section: Arts
Section: Health
Section: Arts
Section: News
Section: News
Health Insurance in Germany is compulsory and sometimes complicated, not to mention expensive. As an expat, you are required to navigate this landscape within weeks of arriving, so check our FAQ on PKV. For our guide on resources and access to agents who can give you a competitive quote, try our PKV Cost comparison tool.
Germany is famous for its medical expertise and extensive number of hospitals and clinics. See this comprehensive directory of hospitals and clinics across the country, complete with links to their websites, addresses, contact info, and specializations/services.
One of the most beautiful squares transforms into a summer stage every year for two days. The Gärtnerplatz Open-Air features a free music and cultural program across three stages, as well as street food from local vendors. On Saturday, the main stage at Gärtnerplatz offers something for everyone,...
No comments yet. Be the first to comment!