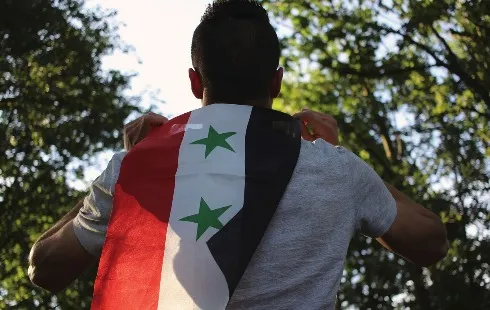
From militant to president, has Syria's new leader Ahmed al-Sharaa gained legitimacy?
Section: News
Researchers at Stanford Medicine have developed a groundbreaking method to utilize the immune system's extensive historical data to aid in diagnosing a wide range of diseases, including diabetes and responses to COVID-19 and influenza vaccines. This innovative approach, termed Mal-ID, leverages machine learning techniques to analyze the sequences and structures of B and T cell receptors, enabling the identification of diseases that are often challenging to diagnose.
The study, which involved nearly 600 participants--some healthy and others suffering from conditions such as COVID-19 or autoimmune diseases like lupus and type 1 diabetes--demonstrated the algorithm's significant success in classifying individuals based solely on their immune receptor profiles.
Current diagnostic methods often overlook the immune system's rich repository of information regarding past infections and vaccinations. According to the researchers, the immune system continuously monitors the body with B and T cells that function as molecular sensors of threats.
By combining insights from both B and T cells, the researchers aimed to provide a comprehensive understanding of the immune system's responses, which could lead to improved diagnostic capabilities for autoimmune diseases and more effective tracking of responses to cancer immunotherapies.
The scientists employed advanced machine learning techniques akin to those used in large language models, similar to technologies behind AI systems like ChatGPT. This method involved analyzing millions of B and T cell receptor sequences to identify patterns that correlate with specific immune responses.
The research team compiled a dataset featuring over 16 million B cell receptor sequences and more than 25 million T cell receptor sequences from individuals with various immune states, including healthy controls and those infected with SARS-CoV-2 or HIV. Their findings revealed that T cell receptor sequences were particularly informative for diagnosing lupus and type 1 diabetes, while B cell receptor sequences were more effective for identifying viral infections and vaccination responses.
The integration of T and B cell data significantly enhanced the algorithm's accuracy in categorizing individuals by their disease state, regardless of demographic factors such as sex, age, or race. This comprehensive approach allows for the identification of subtle variations between diseases that conventional methods may overlook.
By decoding the immune system's record of disease encounters through novel machine learning techniques, the researchers hope to unravel the complexity behind autoimmune diseases like lupus and rheumatoid arthritis. These conditions often present diagnostic challenges, and a more nuanced understanding of their biological diversity could lead to better treatment strategies.
Furthermore, the Mal-ID algorithm may extend its utility beyond the current study's focus. The researchers believe it could be adapted to discover immunological signatures for a wider array of diseases, thus paving the way for new therapeutic targets.
Ultimately, this pioneering work highlights the potential of machine learning to revolutionize disease diagnostics by tapping into the immune system's intricate history. As researchers continue to refine this technology, it may offer significant advancements in clinical decision-making and personalized medicine.
Section: News
Section: Health Insurance
Section: Health
Section: News
Section: Arts
Section: Arts
Section: Politics
Section: Politics
Section: News
Section: Politics
Health Insurance in Germany is compulsory and sometimes complicated, not to mention expensive. As an expat, you are required to navigate this landscape within weeks of arriving, so check our FAQ on PKV. For our guide on resources and access to agents who can give you a competitive quote, try our PKV Cost comparison tool.
Germany is famous for its medical expertise and extensive number of hospitals and clinics. See this comprehensive directory of hospitals and clinics across the country, complete with links to their websites, addresses, contact info, and specializations/services.
Davi Pontes and Wallace Ferreira present Repertório N.2 and Repertório N.3. Two young dancers stand completely naked on stage, rhythmically stamping their feet in energetic patterns that continuously evolve. The physical intensity reverberates through the space, with sweat flying and heavy...
No comments yet. Be the first to comment!