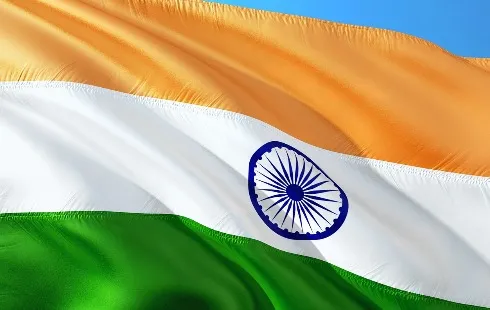
Are war clouds looming over India-Pakistan border?
Section: Politics
An innovative framework utilizing analytics has emerged, aiming to enhance the management of chronic diseases by addressing the socioeconomic and demographic variables that influence patient care. Research conducted by experts at the University of Illinois at Urbana-Champaign highlights how this data-driven approach can lead to more equitable healthcare delivery and improved outcomes for chronic disease patients.
The study, published in the Journal of Operations Management, emphasizes that a predictive and prescriptive model for scheduling patient interactions with healthcare providers can significantly reduce diabetes management risks, particularly among underserved populations.
According to the study's findings, chronic conditions like diabetes require sustained resource commitment and high patient engagement. The diverse characteristics of patient populations contribute to varying health risks, which can directly affect care outcomes. By tailoring healthcare strategies to fit individual demographic profiles, healthcare providers can foster better health results.
The research team, comprising scholars from the University of Illinois, Purdue University, and Lehigh University, aimed to refine diabetes care through a predictive framework designed to allocate healthcare encounters more effectively, particularly for diverse socio-economic groups.
Many patients at high risk for diabetes often do not receive the necessary healthcare encounters, highlighting the importance of personalized chronic care strategies to improve health outcomes. The study points out that mismatches in healthcare access contribute to inefficient resource utilization, exacerbating the inequities faced by chronic disease patients.
Health inequities are a well-documented issue in the United States, where limited resources for chronic disease management create barriers for many patients. Conditions such as diabetes, chronic obstructive pulmonary disease (COPD), cancer, and heart disease require ongoing management; untreated, they can escalate to more severe and costly stages. Early and frequent management of these conditions can help control disease progression and reduce overall healthcare costs.
The researchers analyzed data from over 10,000 diabetes patients collected from a multi-facility clinic across the U.S., supplemented with population-level demographic and socioeconomic data from the U.S. Census. Utilizing machine learning techniques, they aimed to predict individual patient diabetes risks based on previous clinical metrics and socioeconomic indicators, such as income and education levels.
Findings revealed significant disparities in healthcare access, with patients from low-income and less-educated backgrounds, as well as those from predominantly minority communities, being less likely to have regular healthcare encounters, despite exhibiting higher average glucose levels. This disparity underscores the pressing need for risk-sensitive frameworks that can support clinical decision-making processes.
Many chronic care patients hail from under-resourced communities, often lacking consistent contact with healthcare professionals. This gap in care can lead to severe health events, such as heart attacks or kidney failures, as untreated diabetes progresses.
Emergency room visits resulting from untreated chronic conditions impose higher costs and time burdens on both patients and the healthcare system. Regular interactions with healthcare providers can prevent unnecessary emergency hospitalizations, particularly benefiting patients from disadvantaged backgrounds through optimized healthcare allocation strategies.
The implications of this research indicate that healthcare providers can leverage analytics to ensure equitable distribution of limited clinical resources, such as appointment slots. This approach promotes fairer access to chronic care, with the potential to reduce health disparities across populations.
Section: Politics
Section: News
Section: Politics
Section: Health Insurance
Section: Health
Section: Health
Section: Politics
Section: News
Section: Business
Section: Science
Health Insurance in Germany is compulsory and sometimes complicated, not to mention expensive. As an expat, you are required to navigate this landscape within weeks of arriving, so check our FAQ on PKV. For our guide on resources and access to agents who can give you a competitive quote, try our PKV Cost comparison tool.
Germany is famous for its medical expertise and extensive number of hospitals and clinics. See this comprehensive directory of hospitals and clinics across the country, complete with links to their websites, addresses, contact info, and specializations/services.
On Friday, May 9th, 2025, from 19:30 to 22:00, come to Seidlvilla for a captivating concert featuring the Duo.Punto, comprising Elisabeth Maria Krauß on viola and Wolfgang Renner on guitar/arciliuto. The viola, often underestimated as a solo instrument, showcases its rich and dark timbre, which is...
No comments yet. Be the first to comment!