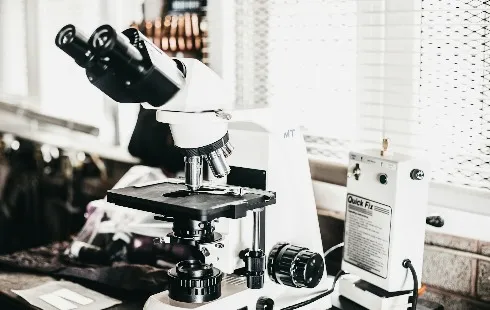
Gonadorelin Peptide: A Gateway to Understanding Endocrine Dynamics
Section: Science
Recent advancements in artificial intelligence have led to the development of a groundbreaking tool designed to assist in the management of atrial fibrillation (AF) by creating synthetic models of fibrotic heart tissue. This innovative approach, pioneered by researchers at Queen Mary University of London, aims to refine treatment planning for patients suffering from this prevalent heart rhythm disorder.
The phenomenon of fibrosis, characterized by the formation of scar tissue within the heart, often arises due to aging, chronic stress, or the AF condition itself. This type of tissue can disrupt the heart's electrical signals, leading to the irregular heartbeats commonly associated with AF. Traditionally, the assessment of fibrosis has relied on specialized imaging techniques such as late gadolinium-enhanced magnetic resonance imaging (LGE-MRI), which provides crucial insights into the pattern and extent of heart scarring, ultimately influencing treatment efficacy.
Standard treatment for atrial fibrillation frequently involves a procedure known as ablation, where small, controlled scars are intentionally created to obstruct erratic electrical signals. However, the success rates of this procedure can vary widely, and effectively predicting which method will yield the best results for each individual patient has posed significant challenges. While AI has shown potential in predicting treatment outcomes, its development has been limited by the scarcity of high-quality imaging data available from patients.
Researchers have tackled this issue by training an AI model on a limited dataset of just 100 LGE-MRI scans from atrial fibrillation patients. This model successfully generated an additional 100 synthetic fibrosis patterns that closely resemble actual heart scarring. These virtual models were then utilized to simulate the performance of various ablation strategies across diverse patient anatomical structures.
The results from this advanced diffusion model demonstrated that the synthetic fibrosis distributions matched real patient data with remarkable accuracy. When these AI-generated patterns were applied to three-dimensional heart models and evaluated against different ablation techniques, the predictions achieved were nearly as reliable as those derived from genuine patient data. Notably, this method ensures patient privacy while allowing researchers to explore a significantly broader range of cardiac scenarios than conventional approaches permit.
This research underscores the emerging role of AI as a supportive tool in clinical settings rather than a replacement for medical professionals' expertise. The aim is not to supplant the judgment of healthcare providers but rather to furnish them with a sophisticated simulator that enables the testing of various treatment options on a digital representation of each patient's unique heart structure prior to the actual procedure.
This project is part of a broader initiative led by Dr. Caroline Roney, focusing on developing personalized 'digital twin' heart models for patients with atrial fibrillation. The researchers expressed enthusiasm about their findings, noting that this advancement addresses the challenges posed by limited clinical data for cardiac digital twin models, thus facilitating large-scale in silico trials and patient-specific modeling aimed at tailoring treatments for atrial fibrillation.
With atrial fibrillation impacting approximately 1.4 million individuals in the UK and the failure rate of ablation procedures being as high as 50%, this innovative technology holds the potential to decrease the necessity for repeat interventions. Furthermore, the AI-driven approach effectively addresses two significant challenges in healthcare: the limited availability of patient data and the ethical imperative to safeguard sensitive medical information.
Section: Science
Section: Health
Section: Arts
Section: Health
Section: Science
Section: News
Section: News
Section: Health Insurance
Section: Health
Section: News
Health Insurance in Germany is compulsory and sometimes complicated, not to mention expensive. As an expat, you are required to navigate this landscape within weeks of arriving, so check our FAQ on PKV. For our guide on resources and access to agents who can give you a competitive quote, try our PKV Cost comparison tool.
Germany is famous for its medical expertise and extensive number of hospitals and clinics. See this comprehensive directory of hospitals and clinics across the country, complete with links to their websites, addresses, contact info, and specializations/services.
The granddaughter of Claire Zachanassian makes a return to Güllen, the impoverished hometown of her late grandmother, for a performance. Having never fully engaged with her grandmother's past, she is eager to finally discover Güllen. The sound of her last name stirs the entire town into action.
No comments yet. Be the first to comment!