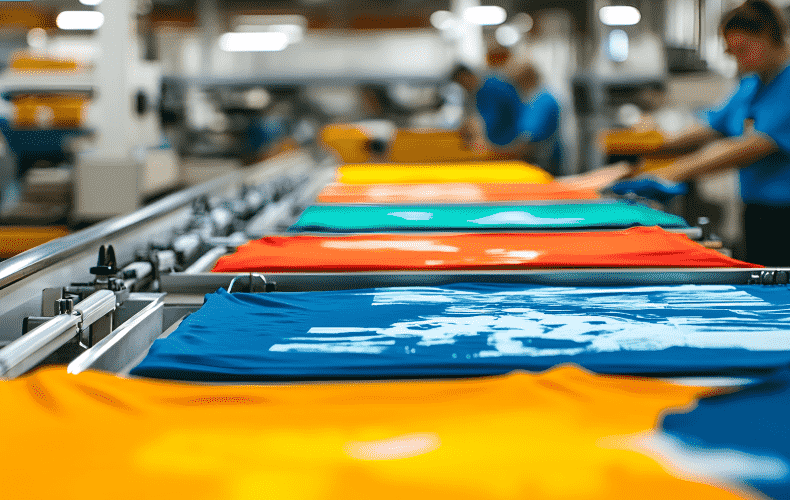
Custom T-Shirts: Last-Minute Gifts They'll Actually Love
Section: Arts
Recent advancements in artificial intelligence (AI) have led to the development of tools capable of assisting clinicians by managing patient triage, gathering medical histories, and even offering preliminary diagnoses. While models such as ChatGPT have shown promise in these areas, their performance in real-world medical settings raises questions.
A study conducted by researchers from Harvard Medical School and Stanford University, published in Nature Medicine, introduces a new evaluation framework known as CRAFT-MD (Conversational Reasoning Assessment Framework for Testing in Medicine) to assess the capability of large-language models in simulating genuine patient interactions.
The study highlights a significant discrepancy between the models' success on standardized medical examinations and their performance in more realistic conversational scenarios. Although the AI models excelled in answering medical exam-style questions, their effectiveness diminished in dynamic, back-and-forth discussions typical of actual doctor-patient encounters. This finding illustrates the necessity for more comprehensive evaluation methods that accurately reflect the complexities of clinical communication.
According to the researchers, the limitations observed in AI performance during these simulated conversations underscore the importance of refining evaluation methodologies. Current testing practices primarily rely on multiple-choice questions derived from medical licensing exams, which often oversimplify the diagnostic process. As stated by one of the co-authors, traditional assessment approaches do not capture the unstructured nature of real-life medical consultations.
CRAFT-MD was specifically designed to address this issue by allowing AI models to engage in realistic dialogues with simulated patients. The framework assesses the models' ability to gather pertinent patient information, make accurate diagnoses, and follow the flow of conversation. The study involved testing four different AI language models across 2,000 clinical scenarios representing common primary care conditions.
The results revealed that all tested models faced challenges in initiating and maintaining effective clinical conversations. They often failed to ask the necessary follow-up questions, overlooked critical patient history details, and struggled to synthesize fragmented information. The accuracy of the AI models was notably compromised when faced with open-ended queries, as opposed to the structured multiple-choice format.
In light of these findings, the research team has proposed several recommendations for AI developers and regulatory bodies. These suggestions include:
Moreover, the study advocates for the inclusion of both AI evaluators and human experts in the assessment process. This dual approach not only enhances efficiency but also minimizes the risks associated with exposing actual patients to unverified AI technologies. CRAFT-MD has demonstrated its capability to evaluate thousands of conversations within a significantly shorter timeframe compared to traditional human-based evaluations.
The researchers express their commitment to continually updating and refining the CRAFT-MD framework to ensure ongoing improvements in AI models' performance in clinical settings. This initiative aims to facilitate the effective and ethical integration of AI tools into healthcare practices, ultimately enhancing patient care.
Section: Arts
Section: Arts
Section: Business
Section: Business
Section: Arts
Section: Health
Section: Arts
Section: News
Section: News
Section: Arts
Health Insurance in Germany is compulsory and sometimes complicated, not to mention expensive. As an expat, you are required to navigate this landscape within weeks of arriving, so check our FAQ on PKV. For our guide on resources and access to agents who can give you a competitive quote, try our PKV Cost comparison tool.
Germany is famous for its medical expertise and extensive number of hospitals and clinics. See this comprehensive directory of hospitals and clinics across the country, complete with links to their websites, addresses, contact info, and specializations/services.
One of the most beautiful squares transforms into a summer stage every year for two days. The Gärtnerplatz Open-Air features a free music and cultural program across three stages, as well as street food from local vendors. On Saturday, the main stage at Gärtnerplatz offers something for everyone,...
No comments yet. Be the first to comment!