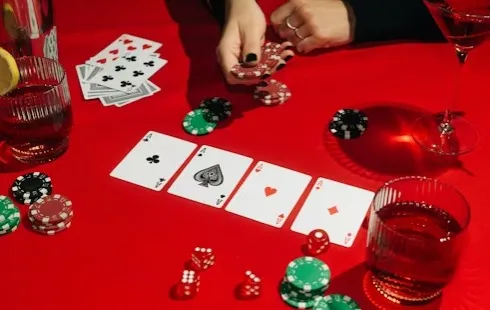
Poker Events That Dominate the International Gaming Scene
Section: Arts
Recent findings presented at the ESCMID Global 2025 event indicate that an artificial intelligence (AI) driven lung ultrasound system significantly enhances the accuracy of pulmonary tuberculosis (TB) diagnosis, surpassing human specialists by 9%.
The innovative ULTR-AI suite processes images captured by portable, smartphone-connected ultrasound devices. This approach offers a rapid, sputum-free, and scalable method for detecting TB, presenting a substantial opportunity to improve accessibility and efficiency in TB screening. Despite a global decline in TB cases in previous years, the incidence of the disease has risen by 4.6% from 2020 to 2023. The World Health Organization (WHO) emphasizes early screening and prompt diagnosis as essential components of its 'End TB Strategy.' However, many countries with a high burden of TB face significant challenges at the diagnostic stage, largely due to the prohibitive costs associated with chest X-ray equipment and a lack of trained radiologists.
Lead researcher Dr. Véronique Suttels highlighted the pressing need for more accessible diagnostic alternatives, noting that the ULTR-AI suite employs deep learning algorithms to interpret lung ultrasound images in real time. This capability enhances the tool's accessibility for TB triage, particularly in rural areas where healthcare workers may have minimal training. By reducing reliance on operator expertise and standardizing the testing process, this technology promises quicker and more efficient patient diagnoses.
The ULTR-AI suite comprises three distinct deep learning models: one that predicts TB directly from lung ultrasound images, another that identifies ultrasound patterns as interpreted by human experts, and a third that leverages the highest risk score obtained from the previous models to maximize diagnostic accuracy.
The study, conducted at an urban tertiary care center in Benin, West Africa, included 504 patients after necessary exclusions, with 192 of these individuals (38%) confirmed to have pulmonary TB. Within this cohort, 15% were HIV-positive, and 13% had a history of TB. A standardized 14-point lung ultrasound sliding scan protocol was employed, with human experts interpreting the images based on established lung ultrasound findings. A single sputum molecular test (MTB Xpert Ultra) served as the reference standard for diagnosis.
The ULTR-AI (max) model achieved a sensitivity of 93% and specificity of 81% (AUROC 0.93, 95% CI 0.92-0.95), exceeding WHO's target thresholds of 90% sensitivity and 70% specificity for non-sputum-based TB triage tests. The model effectively detects recognizable lung ultrasound findings such as significant consolidations and interstitial changes, while its end-to-end deep learning approach captures subtler features that may not be visible to the human eye.
Dr. Suttels noted that a key benefit of the AI models is their rapid turnaround time once integrated into an application, allowing lung ultrasound to serve as a true point-of-care test with substantial diagnostic performance at triage. This capability provides immediate results while the patient remains with the healthcare provider, potentially enhancing patient follow-up and reducing the likelihood of care discrepancies.
Section: Arts
Section: News
Section: Arts
Section: Business
Section: Arts
Section: Arts
Section: Business
Section: Business
Section: Arts
Section: Health
Health Insurance in Germany is compulsory and sometimes complicated, not to mention expensive. As an expat, you are required to navigate this landscape within weeks of arriving, so check our FAQ on PKV. For our guide on resources and access to agents who can give you a competitive quote, try our PKV Cost comparison tool.
Germany is famous for its medical expertise and extensive number of hospitals and clinics. See this comprehensive directory of hospitals and clinics across the country, complete with links to their websites, addresses, contact info, and specializations/services.
Join us for an enchanting evening dedicated to the theme of love, featuring the vibrant sounds of flamenco, rumba, and romantic ballads. Ricardo Volkert & Ensemble will present 'Amor! Amor! Amor!', a celebration of love through passionate guitar melodies, virtuosic cello and bandoneon...
No comments yet. Be the first to comment!